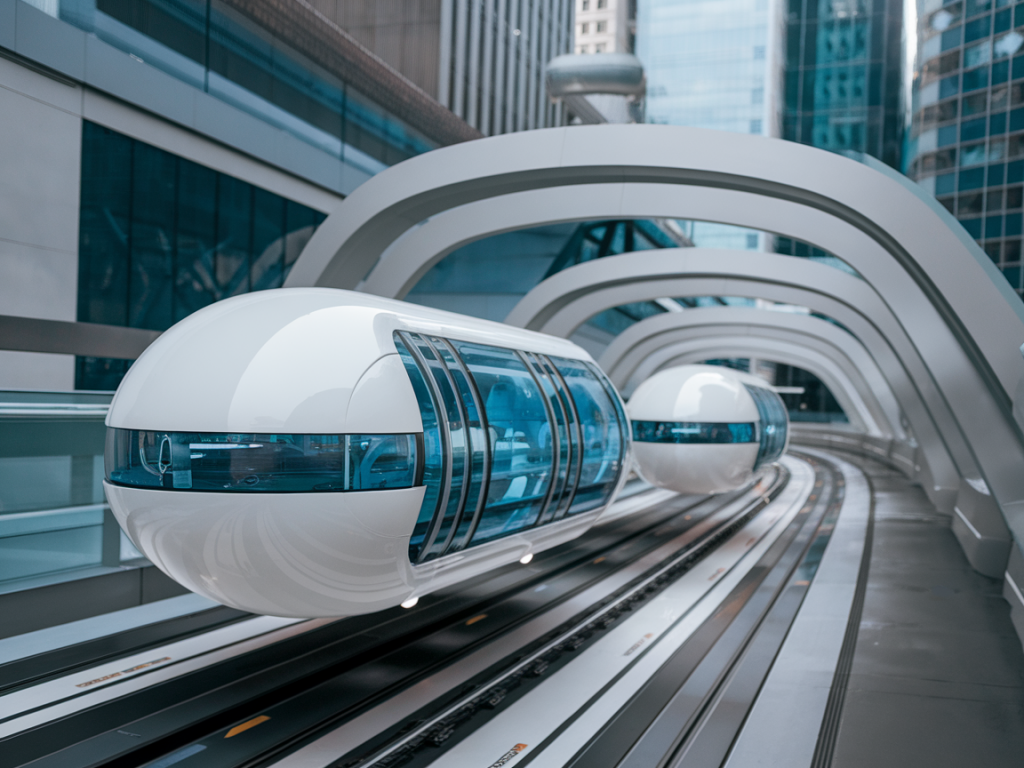
In recent years, generative AI has taken center stage in many industries, but if there's one area where its transformative potential excites me the most, it’s public transportation. As someone deeply passionate about how emerging technologies shape the way we live and move, I find it fascinating to witness how AI—particularly generative models—are beginning to reimagine our future transit systems.
We often think of public transport innovation in terms of new vehicles or infrastructure projects—but what if the brains behind these systems could intelligently optimize and even redesign entire transit networks? That’s no longer sci-fi. Generative AI, with its capacity to analyze, simulate, and propose highly efficient designs, is becoming a key collaborator in the evolution of urban mobility.
What is Generative AI—and How Is It Different?
Before diving into how it’s impacting transit, let’s clarify what generative AI is. Unlike traditional AI, which classifies data and makes decisions based on predefined rules, generative AI can create new content or designs from vast amounts of data. You've probably seen or used tools like ChatGPT, MidJourney, or DALL·E. These models don’t just find answers—they imagine possibilities.
In the transport world, that ability to generate options is game-changing. It means AI can model new bus routes, optimize rail schedules, simulate pedestrian behavior, or even design future bus depots and stations that adapt dynamically to population flows.
Rethinking Transit Planning with AI
Traditionally, public transport planning is a slow and detail-heavy process. It takes years of data collection, stakeholder meetings, and simulations to implement a new bus line or modify a metro schedule. Now imagine handing that process over to a generative AI trained on real-time mobility datasets, geographical statistics, and environmental goals.
Companies like Optibus are already deploying AI-powered algorithms to optimize transit schedules, reducing resource usage while maximizing coverage. In Tel Aviv, where Optibus is based, they’ve worked with local governments to streamline timetables and allocate buses more efficiently based on AI-generated predictive demand modeling.
We’re also seeing initiatives from software firms like CityFormLab at MIT using generative algorithms to design optimal transit-oriented developments. These tools don’t just focus on transit—they evaluate how housing, recreation, and economic clusters feed into demand. In short, AI helps city planners think holistically, beyond vehicles and routes.
Micro-Level Impacts: Passenger Flow and Station Design
One of the most exciting developments is how generative AI is being used to redesign the micro-level elements of transit—things like station layouts, boarding sequence strategies, and pedestrian access points. These may seem minor, but they make a massive difference in user experience and safety.
For example, AI can simulate how crowds move through a busy subway interchange, and then propose entirely new architecture to reduce bottlenecks—something that would take months to analyze manually. Programs designed by firms like Arup or research work at the Fraunhofer Institute rely on generative AI models to craft optimal passenger flows, considering accessibility, emergency evacuation, and intuitive wayfinding.
Generative AI in Autonomous Transit Systems
Another area where generative AI stands out is in real-time routing and system adaptation. Autonomous transit vehicles—whether shuttle pods, driverless buses, or delivery robots—depend on reliable route planning. Generative models can feed these systems with dynamic data inputs like weather patterns, traffic conditions, and current ridership to propose new routes on the fly.
This capability is being tested in smart city labs globally. In Masdar City, Abu Dhabi, one of the world’s most ambitious smart cities, autonomous shuttles powered by AI are piloting new adaptive routing systems that can decide in real-time which roads or schedules are ideal, based on generative predictions of demand spikes or congestion.
AI and Equity in Transit Redesign
One of the most important aspects that generative AI is helping address is transportation equity. Urban transit systems have often neglected underserved neighborhoods, either due to legacy planning or political hurdles. Generative models, by analyzing population density, travel patterns, and demographic data, can make unbiased recommendations for better coverage.
For instance, AI can simulate scenarios like: “What happens if we add a bus line between this low-income area and the industrial park?” or “How does reducing night bus frequency affect women’s safety and accessibility?” These insights empower planners to make data-driven decisions that prioritize inclusion—something I consider an ethical imperative in mobility design.
Collaborating with Human Designers and Planners
Of course, AI doesn’t operate in a vacuum—and nor should it. The goal isn’t to replace human creativity and judgment but to enhance it. I’ve spoken to several urban planners who treat generative AI as a kind of collaborative partner. They set the goals—sustainability, efficiency, inclusiveness—and allow AI to generate solutions that meet those benchmarks in ways humans might not have considered.
There’s a growing toolbox of human-AI collaboration platforms such as Sidewalk Labs’ Delve or Autodesk’s Spacemaker, where planners input design constraints and receive dozens of AI-generated options ranked by performance. While not all of these are transit-specific, their application potential to transport hubs and infrastructure is immense.
Challenges and Ethical Considerations
Of course, deploying generative AI in public transit isn't without challenges. Data privacy, model transparency, and algorithmic bias are significant concerns. If AI-driven models are trained on flawed or incomplete datasets, they could perpetuate inequalities rather than fix them.
There’s also the risk that agencies overly rely on AI without involving the public. I firmly believe that the best mobility solutions emerge from dialogue—between residents, cities, technology, and yes, AI. We must ensure transparency in how decisions are made and that human judgment remains central, especially in matters affecting people’s daily lives.
From the Lab to the Street: Real-World Applications
I’d like to highlight a few inspiring pilot projects that show generative AI is not just theory—it’s already reshaping our streets:
- Helsinki, Finland: The city has used AI-based simulations to redesign bus networks that reduce transit time by 15% while improving coverage in the outer suburbs.
- Sydney, Australia: Transport New South Wales deployed generative AI to test traffic-light synchronization across the city, leading to noticeably improved bus punctuality.
- Hamburg, Germany: Their "Reallabor" project uses AI to dynamically propose how to reduce private car use and optimize shared mobility services.
Every time I see one of these initiatives launch, I’m convinced that the fusion of AI and urban mobility is just beginning. As these tools spread and mature, I believe we’ll develop transit systems that are not only more efficient—but reflective of our collective goals for how we want future cities to thrive.